The Mosquito Simulator
- Arbel Vigodny
- Dec 5, 2019
- 2 min read
Updated: Jun 4, 2021
Mosquitoes are a spatial phenomenon. Your house may be overrun with buzzing mosquitoes, while your neighbor, living only 300 meters away, is resting unbothered in his backyard. That is not to say that mosquitoes are lazy. In fact, they spend a lot of their time flying, but usually without a specific direction or destination. Ninety percent of mosquitoes of certain species will never fly beyond 1km from where they were born. This is why some parts of a village may be “malaria hotspots” while others are not.
How can we use this fact to save people from malaria? First we need to identify the hotspots. There are a few ways to do that: we can analyze malaria cases if we know the exact location of patients; we can analyze satellite imagery to understand which areas are more suitable for the formation of water bodies; and we can use data about the actual location of water bodies. The next step, after defining a hotspot, is to come up with a suitable intervention strategy. For example, in the dry season, when water bodies are fewer, we can spray houses near water bodies or simply install screens in their windows.
Although these strategies are simple and usually very inexpensive, they are currently not in wide use. The reason for this is that they require a particular understanding of each village, and therefore are difficult to deploy on a large scale. In addition, it is not always easy to predict the impact of combining different interventions, whether they will complement each other or rather create redundancy. Epidemiological models are designed to predict the impact of interventions on a large scale, but spatial models are needed to do the same at this high resolution.
Our simulator addresses these problems. It combines a spatial model with weather analysis and real world data on the location of water bodies and houses. Unlike many spatial models that test theoretical hypotheses on theoretical locations, our simulator is designed to work in real, and specific, villages and towns.
The simulator not only predicts the impact of specific interventions, but also recommends the best one. Here’s the thing: even for a single village there are millions of possibilities — millions of combinations of houses and water bodies to spray at different times and sequences. Sifting through these possibilities, even for a computer, is difficult. Our AI deals with this complexity by running coarse searches through random possibilities, gradually zeroing in on the ones with the strongest impact per cost.
The simulator is built to constantly improve as more field data is collected for the algorithm to train on. As larger field operations using the Zzapp mobile app are launched, the data will become more reliable and applicable to a wider range of environments.
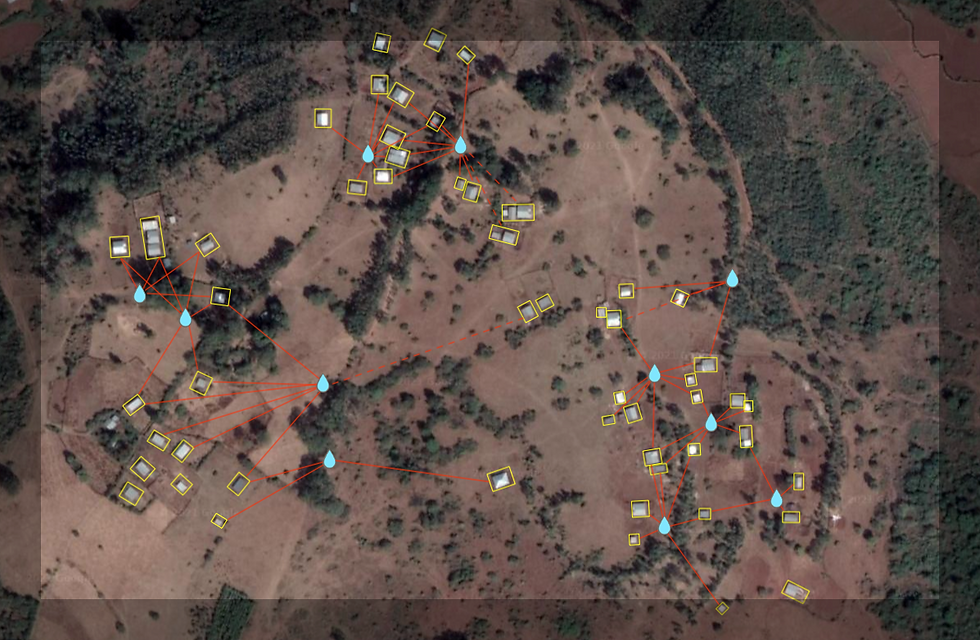
コメント