AI and Operations — Opinion
- Arbel Vigodny
- Jun 6, 2019
- 3 min read
Updated: Jun 4, 2021
By Arnon Houri Yafin
In recent years, there is a growing interest in applying AI to a variety of traditionally low-tech industries, and this has included revolutionizing the very structure of operations management. Services like food delivery are being decentralized, while other processes, such as shipping, are becoming centralized, leading to significant optimization of resource and manpower utilization.
AI occasionally provides surprising insights, but its main contribution is the improvement of operations as they scale up. Without AI, managers are limited in the amount of data they can process, and are thus unable to optimize every aspect of an operation at once.
For example, there have been many efforts to optimize supply chain management using AI. The first phase of such an endeavor is digitization — IT solutions that help employees keep track of what they are doing, allowing smart algorithms to use the data to make predictions. These IT solutions are already prevalent, with companies using systems such as ERP (Enterprise Resource Planning), MES (Manufacturing Execution System) and PPC (Production Planning & Control). AI algorithms that are fed by different agents across the supply chain (e.g., manufacturer, forwarder, warehouse) will be able to improve planning of delivery times and prediction of shortages in specific products at the stores. If this data is further correlated with data on customer demand, it can be a powerful tool for companies.
An additional use for AI in operations is promoting collaboration between different users and platforms. Companies like Uber correlate data on supply time from nearby restaurants with traffic data to optimize food delivery. However, as always, the crucial condition for scale of services is the human factor. Companies analyze behavioral data to reward their best users, such as taxi drivers who take on less profitable rides. They also use AI and data to find gaps in users' understanding of their technology, and to detect fraud — users that do not really do as they claim. Here again, the more the users digitize what they do, the more efficient the technology becomes.
A common aspect shared by all AI used for operations is the importance of micro-timing. Unlike algorithms that, for instance, recommend prices for cheese based on overall demand, operation algorithms must coordinate service provider and service receiver on a minute by minute basis; when it’s raining, you need your taxi NOW.
Another interesting aspect of AI used in operations is anomaly detection. Certain rare events can cause a lot of damage for an operation. Because such events may be too complex for algorithms to manage, AI engineers focus on devising efficient alerting systems to ensure quick detection, or, as it is known in the field, to decrease the mean time of detection (MTTD). These algorithms operate like police officers who sense that something is amiss, increasing their presence on the streets even before realizing what the problem is.
Building AI for operations is a very application-specific task. Occasionally, the factors to be taken into account are similar, and differences are small enough so that algorithms can adjust to make predictions across different applications. Often, however, the difference is so big that you must change not only the algorithm, but also the questions that you ask it.
At Zzapp Malaria we deal with very unique problems, but the basic rules of the field apply to us. We harness innovative approaches and AI algorithms to improve Anopheles mosquito control operations. The first part is to digitize more data, including mosquito prevalence, malaria cases in hospitals, usage of the spraying materials, and above all — operational field data: which worker mapped which areas and sprayed which water bodies, and when. This time and location-specific data is digitized and fed into the system through our mobile app, which accompanies workers through the various stages of the operation. The second part is understanding what the important questions are — is it the timing of the spraying activities? Their intensity? Their location? Lastly, we focus on tracking: raising managers' attention to problematic events. By following the general rules of AI for operations, we are able to simplify the process and provide AI-based solutions for our collaborators in the field.
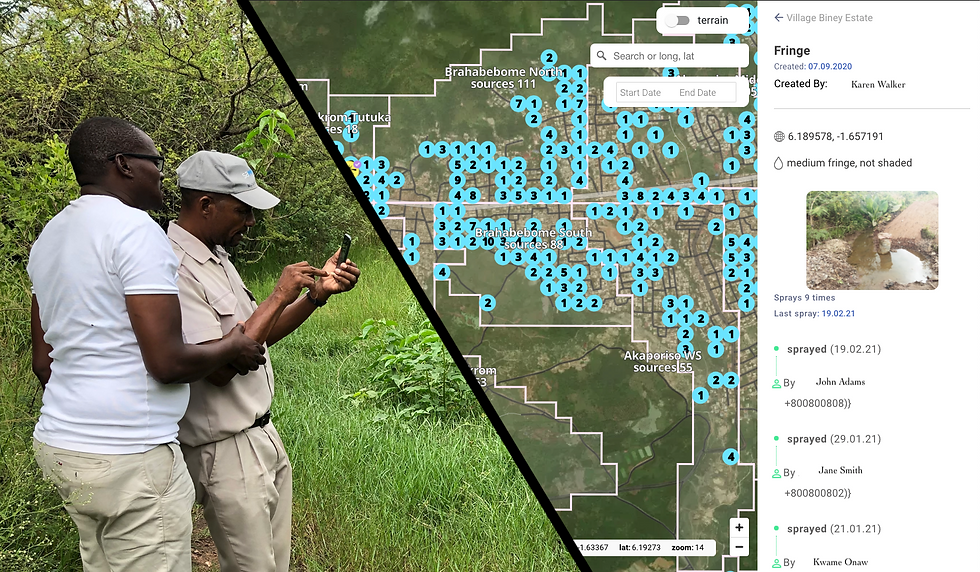
Comments